Integrating SAP S/4HANA, Siemens OpCenter EXPR and Siemens OpCenter RD&L.
How to release a warehouse full of packages of unknown quality and avoid delayed delivery at the customer? Well, you just know. In this case, Empiriqation was asked to achieve a fast quality delivery to the customer. The key enabler for this, is: data integrity. In this case a few principles were applied.
One given, is that the master data between S/4, manufacturing and the scientists / research center is well related to each other. And because each package should contain top-grade quality content, it is essential to have a good statistical scatter of laboratory samples. Data integrity makes sure that the final quality management decision, to ship a finished good to a customer, is a correct decision based on first-hand/original facts throughout the manufacturing processes. A typical diagram to summarize all these original facts in the processes, is the fishbone diagram like below.
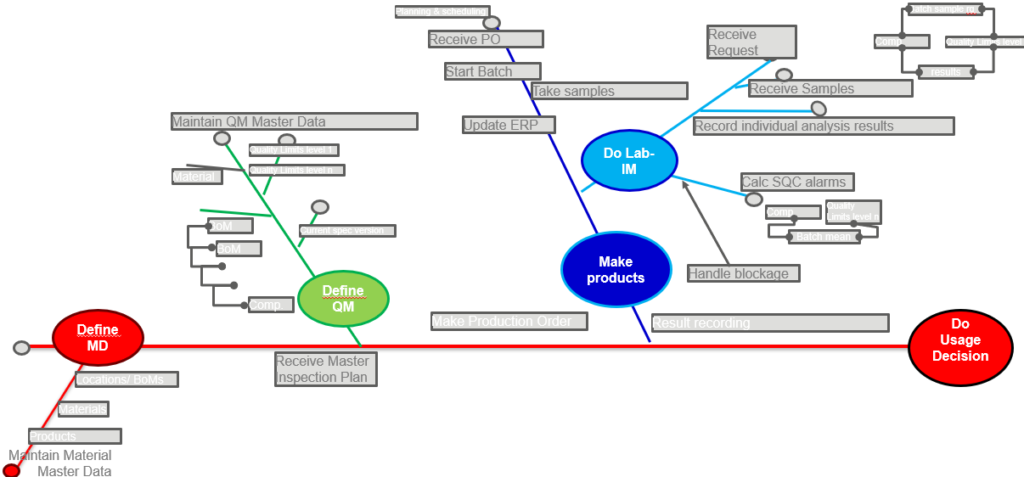
In the fishbone-diagram, the break-up of the final goal from right to left is demonstrated. Each process that is the origin of key-data elements is separated from the main and contains key-data origins from themselves.
Features of solutions make them suitable in the fishbone diagram:
- ERP Usage Decision feature is applied on inspection result data;
- MES Bill-of-Process feature sends inspection results at completion;
- LIMS scientific statistical calculations produce reliable results;
- Spec Mgt for continual inprovement of BoM, quality and safety;
- ERP Master Data for nomenclature and QM-relevant inventory;
In this case, the solutions where designed based on S4HANA for ERP, Siemens OpCenter EXPR for MES and Siemens OpCenter RDnL for LIMS and Spec Management. The processes where rolled-out on a global scale for the first phase: Europe & America’s. After one pilot plant, the data-integrity solutions absorbed the products of over 20 locations like naturally (I can’t emphasize enough how strong information based on data-integrity really is – if the model is right it rolls hill down if it isn’t right it rolls hill up). This phase took more than a year.
Training on data-integrity: everyone on the production line should know the basic context of their work to have direction over the daily task. Taking samples for quality control and process control isn’t the task of the Lab or Engineers because the origin of this data is the first section of the blue make-products process that they are operating on themselves. The Lab process (light blue) is the origin of quality results only based on the data-input from red, green and blue. So line-operator himself should facilitate sample-analysis over the manufacturing operations to guarantee a high quality product is coming off the line. The main purpose of data-integrity training is to propagate the value of critical thinking: how does the operator know certainly that the line is operating correctly and safely? – and include the help of the Lab and the engineers in this open question.
The lab analysts receive samples from many lines and don’t need to know at which moment on a given day a line is scheduled for maintenance or active. This information could be provided to them but from workflow point of view they don’t have a need-to-know. So the IT solutions are mostly not arranged in such a way because the solutions don’t often provide such features and don’t need to. Vice-versa, a statistical update on inspection characteristics and limits is not information that the Lab must share with the operations. These statistics are original in the light-blue process line based on a scientific background.
Finally some multi-dimensional figure to indicate the different organization models and the integration of the data that originates there. Next case, I hope we can make some improvements by starting in a more concept-centred way. There are considerations to apply blue to Spec Mgt, Yellow to ERP, Red to MES and that makes the green part fluently for Research and Innovation based on science and ethics through the Labs using QM.
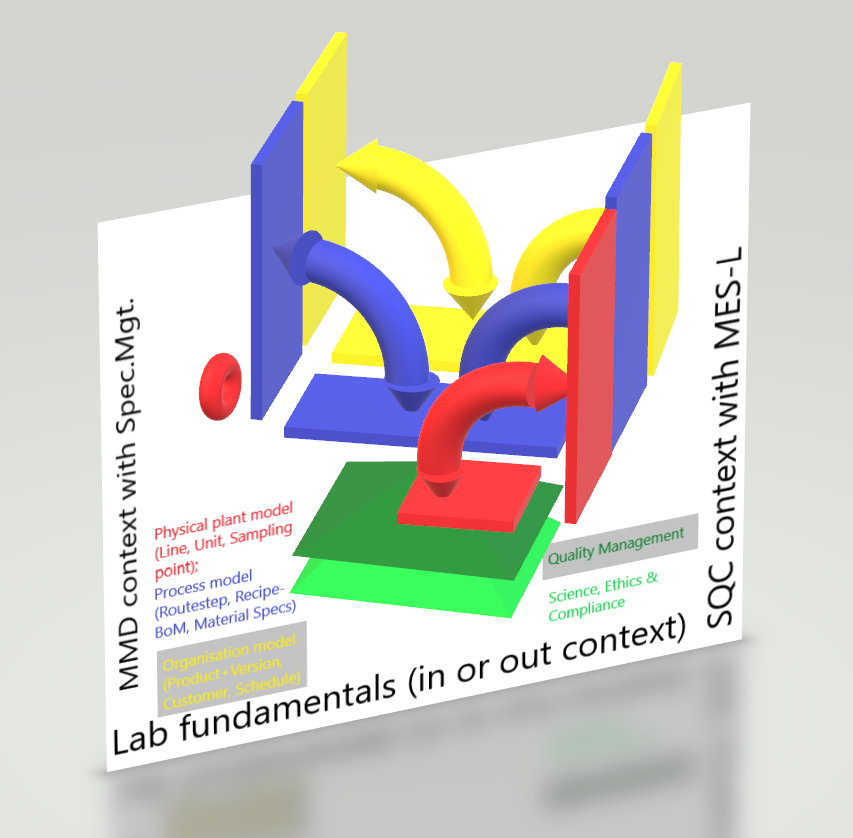
Conclusion: the case was completed and the solution has landed and expanded over EU and America’s. Data-integrity is relatively new (after 2016) and there were shortcuts taken for the sake of bad habits. The value of data-integrity is appreciated and returned in a culture of critical thinking, therefor training is essential.
Additional opinion: selecting people for the training is mostly done in a wrong way. There must be an eye for talent and this is input for the training and the internal culture of critical thinking. In each model in the 3d-picture there are different talents needed. We encountered hesitation to train young people to bypass seniors based on status and fear of loss of control. However this hesitation is based on irrational influences which sabotages the new culture of critical thinking and therefor its value isn’t appreciated to the fullest. At the end, the talents will find a way to develop itself, despite the irrational resistance. The more freedom talents have to take the position where they belong, the more beneficial it is for the organization.